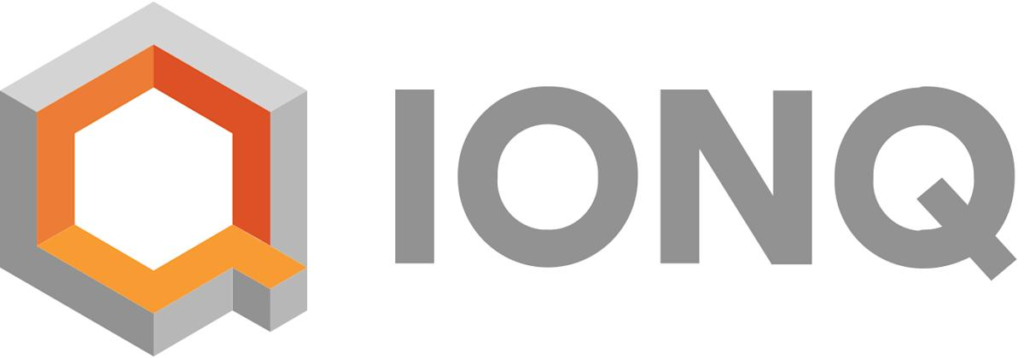
IonQ has announced two new research achievements demonstrating how quantum computing can augment artificial intelligence workflows, particularly in the domains of language modeling and materials science. In one effort, IonQ researchers integrated a quantum machine learning (QML) layer into a pre-trained large language model (LLM) to improve its fine-tuning on sentiment classification tasks. The hybrid quantum-classical architecture outperformed classical baselines with similar parameter counts and showed promise for improved accuracy and energy efficiency as the problem size scales beyond 46 qubits.
In a second initiative, IonQ collaborated with a major automotive company to apply quantum-enhanced generative adversarial networks (QGANs) for image augmentation of steel microstructures. These synthetic images, derived from a hybrid quantum-classical pipeline, achieved higher quality scores in up to 70% of test cases compared to classical GAN baselines. The project addresses a key limitation in industrial AI: the scarcity of high-quality, domain-specific datasets for training models that guide material optimization.
Both projects illustrate IonQ’s broader strategic focus on near-term, utility-scale applications of quantum computing in AI. The company continues to explore integrations with Ansys for quantum simulation in engineering and is also partnering with AIST in Japan to advance quantum-AI research. These developments reinforce the role of hybrid quantum systems in enhancing AI capabilities across sectors such as natural language processing, manufacturing, and scientific computing.
Read IonQ’s full announcement here, and explore the technical details in the accompanying research papers on quantum LLM fine-tuning here and QGAN-based image augmentation here.
May 1, 2025