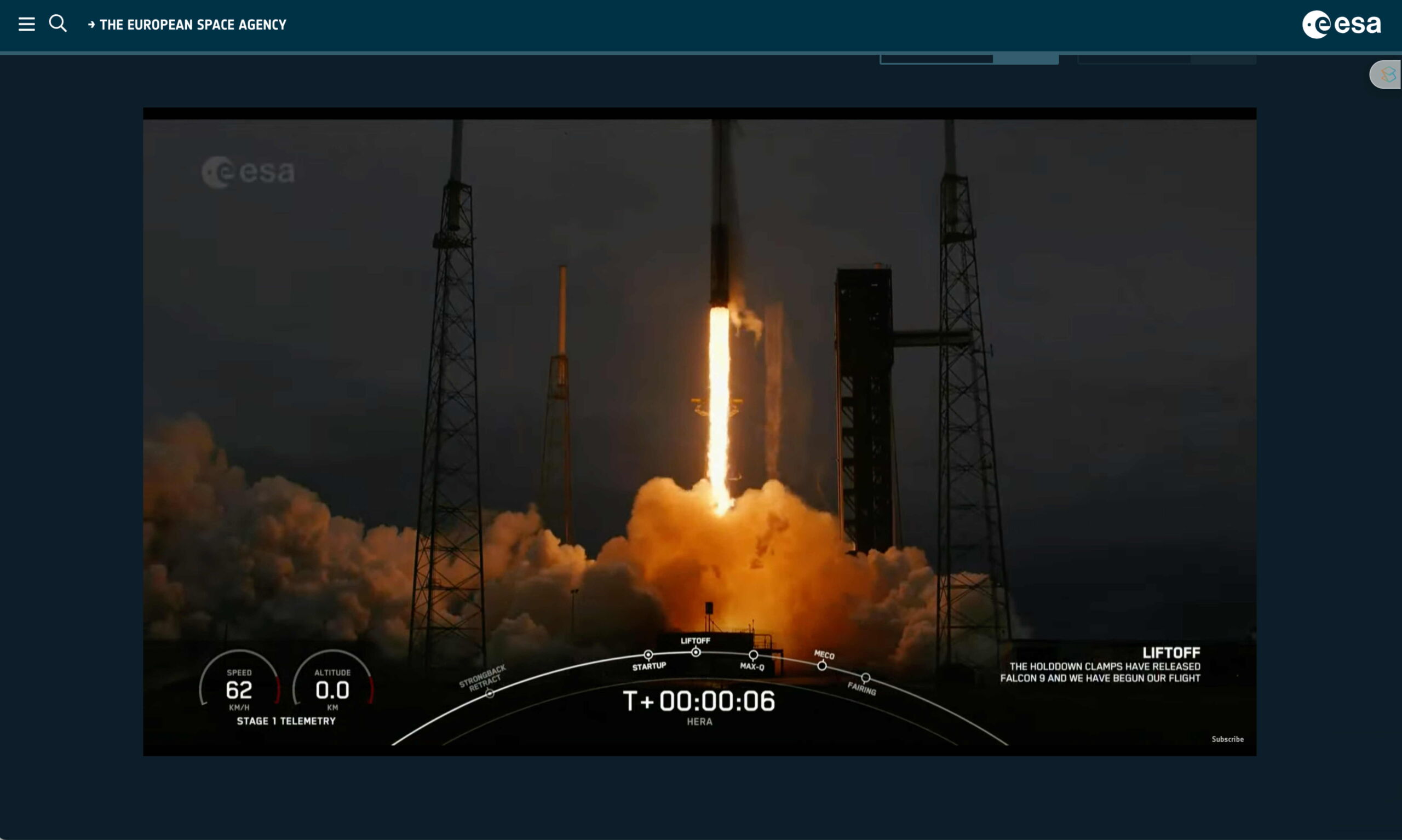
by Amara Graps
Quantum in Computational Fluid Dynamics (QCFD) has emerged as a hot research area in quantum computing in recent years. You may be familiar with Rolls Royce’s Collaborations and Use Cases, but there are other lesser-known examples implemented in pilot projects today as well. As CFD is widely utilized in the automotive, aerospace, civil engineering, wind energy, and defense industries (Dalzell et al., 2023), these are grouped together as ‘Advanced Industries’ in GQI’s Use Cases categories in the Figure below.
One example of the crucial role of classical CFD in SpaceX’s rocket design, testing, and optimization is the near-perfect performance of launches, such as the one that put the ESA Hera asteroid defense mission on its interplanetary route on October 7 (header figure).
Is CFD ready for quantum?
A variety of indicators says ‘Yes’!
This 3-part series gives guidelines to:
- Part 1) the Quantum CFD Literature,
- Part 2) the QCFD Use Cases algorithm implementation: Linearization-Forward,
- Part 3) the QCFD Use Cases algorithm implementation: On the Native Hardware with Lattice-Boltzmann
Quantum CFD Scientific Literature
My primary source for algorithm research, Dalzell et al., 2023, has an excellent section on computational fluid dynamics (CFD); I’ve partially replicated the references here. Typically, CFD implements a Navier-Stokes equation simulation. While most simulations concentrate on air or fluid movement on solid objects, it’s important to mimic other processes as well, such foaming. Large CFD simulations, which are sometimes run at petaflop speeds, require millions of CPU cores. Some approaches to quantum algorithms are listed in the following Table. I’ve grouped the papers: Introductions, Linear Algebra-Forward, and Native Hardware. Most are Linearized with good results; the Native Hardware algorithm is in a special class. The reason for this grouping will be clear in Part 3.
QCFD Introductions
- Bharadwaj et al., 2020 is a very good broad introduction to quantum scientific computing, then a focus on CFD implemented with several different quantum devices. Despite its 2020 publication date, the core algorithms and modality descriptions haven’t changed. I would start here to begin.
- In the two articles by Gaitan (2020, 2021), the Navier-Stokes equation’s steps, their discretization to convert them from PDEs to ODEs, and subsequently recommended techniques to execute on a quantum computer, are described. Then the Navier-Stokes ODEs are solved using his recommended approach, the Kacewicz quantum ODE algorithm, which is based on the quantum amplitude estimation algorithm (QAEA). Since the QAEA only has one role- estimating his equation (16)- it can be replaced by newer algorithms that have emerged in this rapidly developing field while he was writing his paper.
A New Middle Ground
In GQI’s Quantum Algorithms Outlook report, GQI writes about ‘a new middle ground’:
While VQE (low depth for NISQ applications) is often viewed in contrast to QPE (high depth requiring FTQC), interest has grown in other techniques that might offer a middle ground.
The QCFD algorithms fit into this category, for both classes: Linear Algebra-Forward and Native Quantum. See the list of algorithm summaries how they adapt to the NISQ devices, successfully.
QCFD Algorithms: Linear Algebra-Forward
- Gourianov et al., 2021 use tensor network theory, a quantum-inspired mathematical framework for expressing and manipulating high-dimensional data by breaking it down into networks of lower-dimensional tensors. They apply it to the development of a structure-resolving turbulent flow. Compared to direct numerical simulation, they find that this technique allows correct solution of the incompressible Navier-Stokes equations while significantly reducing the number of parameters needed to represent the velocity field, achieving a compression ratio of over one order of magnitude compared to direct numerical simulation.
- Kiffner and Jaksch, 2023, apply a tensor network algorithm, here to reproduce the stationary state data for the lid-driven cavity at low Reynolds numbers. The algorithm captures the short-time dynamics of flow in both lid-driven and doubly driven cavities. The physical model is discretized using FEM, then the linear equations are solved for an initial training set of inputs. The training stage uses an appropriate quantum algorithm to solve the system of equations exponentially faster. Then another model is applied to simplify the original problem, allowing future simulations to solve it conventionally without a quantum solver.
- Jaksch et al., 2022 apply variational quantum algorithms (VQAs) to CFD (VQCFD). With a demonstration of these algorithms applied to Burgers’ Equation, the study illustrates how quantum computing can provide a quantum advantage over classical methods, especially in solving nonlinear optimization problems.
- Bosco et al., 2024 describe their Hybrid Quantum-Classical Finite Method (HQCFM) to show high accuracy in solving the 2D, transient, incompressible, viscous, non-linear coupled Burgers’ equation, with results comparable to classical solvers for large systems up to 2048 mesh points. Additionally, the HQCFM exhibited scalability and consistency, indicating that a 30-qubit quantum computer could outperform Exascale computers in handling complex real-world simulations.
- Oz et al., 2022 presents a QCFD solver specifically designed to solve Burgers’ equation (BE). It adapts a quantum algorithm for partial differential equations to BE, demonstrating its effectiveness in handling flows with and without shockwaves. The quantum simulation results were validated against both an exact analytical solution and classical CFD solver results, showing excellent agreement and comparable error rates.
- Lapworth et al., 2022 presents a hybrid quantum-classical Computational Fluid Dynamics (CFD) methodology that utilizes the Harrow, Hassidim, Lloyd (HHL) algorithm to solve linear systems of equations relevant to CFD problems. The study includes tests on 2D lid-driven cavity cases, demonstrating that hybrid solutions can match classical solutions with high fidelity, particularly for smaller mesh sizes.
- Succi et al., 2024 assesses the feasibility of using the functional Liouville formulation for ensemble simulations of fluid flows on quantum computers, highlighting the need for hundreds of logical qubits to achieve practical simulations. The study concludes that the Liouville approach is preferable for ensemble simulations, contingent on achieving reliable qubit performance.
QCFD Algorithms: Quantum Native
- Itani et al., 2023 describe a full quantum algorithm for the Lattice Boltzmann method for simulating fluid flows, which is the only one that implements both the streaming and collision sub steps as unitary operators with an efficient number of qubits. They use Hamiltonian simulation underpinning the method and demonstrate that it employs qubits that scale logarithmically in the flow Reynolds number but gates that scale only polynomially.
- Li et al., 2024 demonstrates that quantum solvers, specifically through the lattice Boltzmann equation, can effectively simulate turbulence, achieving logarithmic scaling in the number of degrees of freedom, which is a significant improvement over the polynomial scaling of classical algorithms. The study emphasizes the need for further development to address complex boundary conditions and heterogeneous systems to fully realize the potential of quantum algorithms in practical applications.
Quantum Computing Use Cases Live Tracker
From the above descriptions, many studies linearized the Navier-Stokes equation to find components that could run on the quantum device, while other components run on the classical device.
In GQI’s framework for Use Cases, quantum algorithms for ‘Linear Algebra’ would fall under the Quantum Linear System Solver (QLSS), which we described previously in QCR: Quantum Algorithms for Solving Differential Equations. We will proceed to describe QCFD Use Cases in Parts 2 and 3.
If you are interested to learn more, please don’t hesitate to contact info@global-qi.com.
October 8, 2024