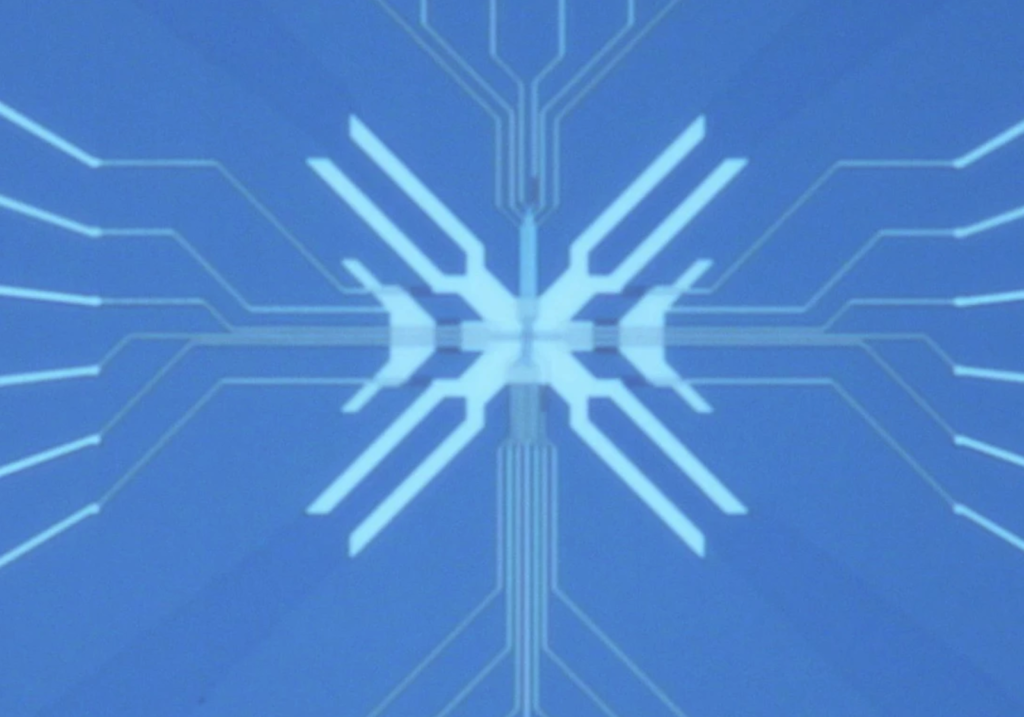
by Amara Graps
Scaling Semiconductor Spin Qubits
This article begins with a scaling message,
We need these machine learning techniques to accelerate the scaling of these quantum devices.
spoken by Natalia Ares (Professor, University of Oxford), at the panel discussion: Challenges When Going Towards 1000 qubits. The June 14, 2022 panel was part of the three-day Quantum Technology User Meeting 2022 in Munich, a quantum technology cluster. See our QCR article about Germany’s Quantum Technology Ecosystem, here. This panel consisted of a group of quantum technology leaders of companies that sell quantum control hardware, superconducting or photonic quantum computers, or quantum machine learning software. The panel was facilitated by Sadik Hafizovic, CEO of Zurich Instruments.
To place Natalia Ares’ message in context, embedded in every quantum machine learning topic is the two-sided question:
- What can AI do for quantum technologies?
- What can quantum technologies do for AI?
As Ares’ group applies machine learning to the control of quantum devices in real-time, she was addressing the first of the two-sided questions. Her theme for the challenges panel was: “If one is to scale to 1000 qubits, then it is not possible without machine learning.”
Her contributions to that panel were a perfect lead-in to her one-hour detailed presentation: Machine Learning Based Control of Quantum Devices at the same 2022 meeting about her group’s work to optimize qubit operations. Her group works with qubits in several modalities: ion traps, superconducting, and semiconductor spin qubits, which led to a spin-off company: QuantrolOx. GQI’s QCR interviewed QuantrolOx’s CEO: Vishal Chatrath in 2023, and we wrote a company-focused Focus Report on the Midstack, which we’ll describe shortly. In Natalia Ares’ presentation she focused on the latter qubit: the semiconductor spin qubits.
Steps for the Development of the Machine Learning Algorithms
Ares described how their algorithmic approaches transitioned from No Deep Learning, because there was not enough data, to Deep Learning approaches, as more data was acquired. Their algorithm development used the steps, as seen in the next Figure.
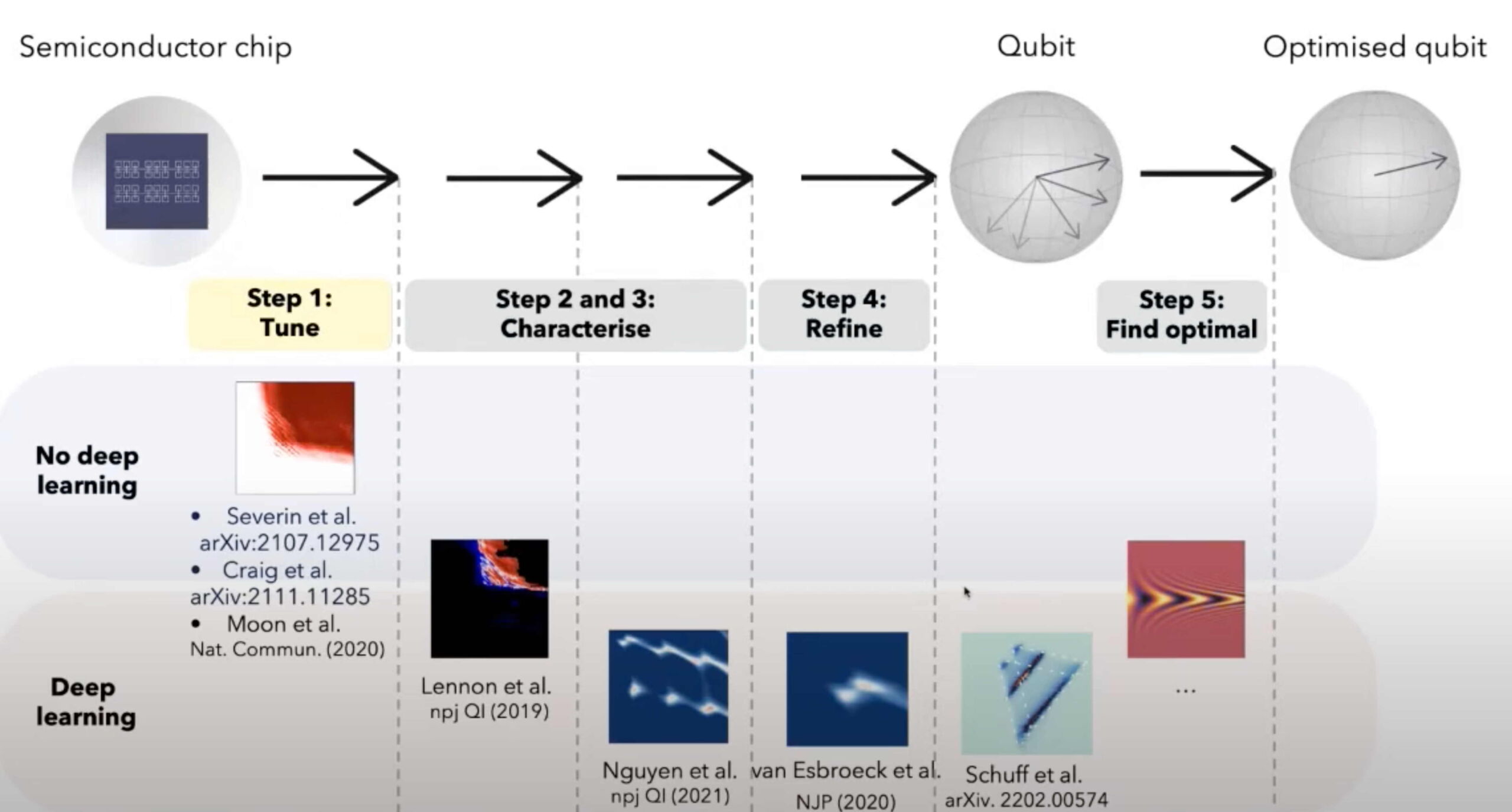
The necessary steps are:
Step 1: “Tune” Super coarse tuning step.
- Studying the gate voltages of one compared to another.
- Algorithm needs to represent multidimensions.
- Investigate and choose candidate locations.
Step 2 and Step 3: “Characterize”
- Deep Reinforcement Learning.
- Certain actions (e.g., gate voltages) have some rewards.
- Characterization in these steps
Step 4: “Refine”
- Initial Scan in real-time
- Score (use a neural network)
- Choose gate voltages
- Perform next scan in real-time
Step 5: “Find Optimal”
- Pauli Spin Blockade (spin to make a qubit)
Machine Learning in 2024 for Semiconductor Spin Qubits
How far have researchers come in using machine learning for semiconductor spin qubits? Recent results by an Australian-led group from the University of New South Wales, Diraq, University of Sydney, Simon Fraser University, Leibniz-Institut für Kristallzüchtung, VITCON Projectconsult, and from her Oxford group, by Huang et al., 2024: High-fidelity spin qubit operation and algorithmic initialization above 1K addresses one of the challenges of these semiconductor spin qubits as these qubits scale:
These advances overcome the fundamental limitation that the thermal energy must be well below the qubit energies for the high-fidelity operation to be possible, surmounting a main obstacle in the pathway to scalable and fault-tolerant quantum computation.
Their research suggests that machine learning techniques can be efficiently implemented directly inside the FPGA for real-time advanced calibration and automation of the initialization protocol. Machine learning for quantum, enters their research:
- Machine learning is utilized for SPAM (State Preparation and Measurement) error analysis by leveraging increased statistics from multiple measurements. This involves using a hidden Markov model (HMM) to describe the series of measurements and infer the underlying spin states.
- The Baum-Welch algorithm, a machine learning technique, is employed for expectation maximization to fit the HMM parameters, which helps in quantifying the uncertainty in these parameters.
- Machine learning aids in reconstructing the probabilities of state changes during each readout cycle, enhancing the fidelity of the algorithmic initialization process.
Machine Learning in the Quantum Computing Midstack
In the GQI’s vision of the Quantum Tech Stack, it is in the Quantum Computing Midstack, where machine learning techniques for real-time control of semiconductor spin qubit computers, enters.
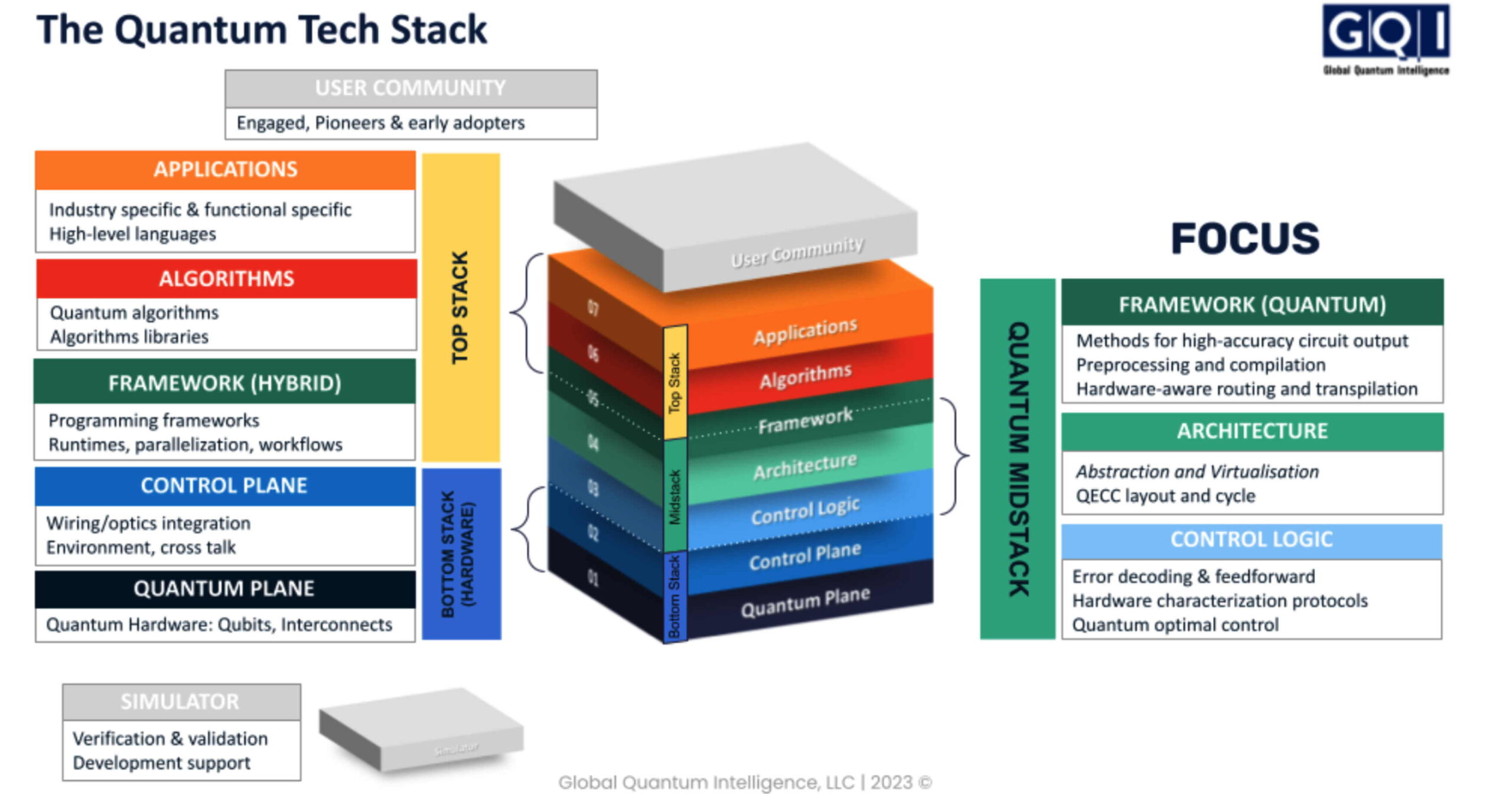
In GQI’s Quantum Computing Midstack Report (*) sponsored by QuantrolOx, the vital conduit between qubits and algorithms is highlighted with its complexities. Along the way, the Report shows where machine learning techniques can provide optimization techniques, error correction protocols, and error suppression strategies. QuantrolOx’s mandate is automating the critical area of qubit control and quantum optimal control. They have a collaborative strategy, for example: see their Spring 2024 announcement with Zurich Instruments, that aims to expand beyond superconducting qubits to be compatible with silicon spin, NV diamond centers and subsequently ion-trapped and neutral atoms qubits.
(*) GQI with QuantrolOx’s 63-pg Quantum Computing Midstack Report decodes the complexities showing the Midstack layers: Error mitigation, Optimization, Error Correction, the Control Logic. The Quantum Computing Midstack structure is further decoded, showing you its Functionality and Layers. The Report includes Midstack Developments to watch. The Midstack’s role in the evolution of quantum computing applications is tackled with Timelines for quantum algorithms applications. Finally, a Quantum Midstack Market Analysis is provided. If you are interested, please don’t hesitate to contact [email protected].
September 26, 2024