by Amara Graps
Cancer Use Cases
For social impact, there is no greater human health challenge than cancer, for Use Cases of today’s quantum technology.
Solving cancer is a multidisciplinary grand challenge for the past several decades, and a natural fit for the HPC community, as seen in this 1992 USA, HPC example. This grand challenge has grown to cross international borders, for example, the Cancer Grand Challenges Org from the Cancer Research UK and the National Cancer Institute in the US.
An inherent ‘multidisciplinary’ and ‘HPC’ theme of any human challenge inspires a quantum approach, as seen in last year’s Welcome Leap pool of winners for their Quantum for Bio initiative. One of the Quantum for Bio initiative winners is a team from Infleqtion, the University of Chicago, and MIT, with a project to use artificial intelligence (AI) and quantum algorithms to develop personalized diagnostics and treatments for cancer. How is their work progressing?
Cancer Applications: Broadly
First, the team’s broad view. Tomesh et al., 2024 in a blog post at ACM SigArch: Co-Design of Hybrid Quantum-Classical Applications in Multimodal Cancer Research highlighted the need for multidisciplinary cooperation to realize the full, long-term potential of quantum computing for oncology, which includes mapping complete cancer genomes and regulomes, fully simulating molecular dynamics for drug formulation, and using quantum sensors to monitor tumors. These approaches were elaborated in the team’s Nature paper: Ramesh et al., 2024, in Quantum computing for oncology, where their Table 1 lists the molecular modeling, genomics, quantum imaging and quantum sensors applications with a useful column for Methods and Techniques.
Table 1 – Quantum Computing Approaches and Advantages for Oncology Applications
Approach | Advantage | Methods & Techniques | Oncology Application |
Molecular Modeling | • Quantum computers can encode an accurate representation of molecular systems using a polynomial number of qubits, whereas a classical computer would require an exponential amount of memory to store the same representation. • Molecules and quantum computers both operate under quantum mechanical principles, enabling efficient simulations of classically intractable systems. | 1. Variational quantum eigensolver (VQE) 2. Hamiltonian simulation 3. Quantum phase estimation 4. Quantum machine learning (QML) | 1. In silico modeling of small molecules and/or proteins for targeted therapies 2. Characterization of off-target effects for novel therapeutics |
Genomics, Transcriptomics, and Multiomics Analysis | • An exponential amount of classical data can be stored in a polynomial number of qubits using amplitude encoding, which may offer increased efficiency in analyzing complex multiomic datasets representing -omic profiles. • Quantum machine learning models achieve at least a quadratic memory separation over classical machine learning models for finding patterns in genetic data with correlations across long genomic distances in the genome or between multiple -omic markers by exploiting quantum contextuality. | 1. Grover’s search algorithm 2. Quantum annealing 3. Quantum approximate optimization algorithms 4. Quantum machine learning (QML) | 1. Multiomics characterization of novel tumor subtypes 2. Sequence-based analysis of long-range genome relationships |
Quantum Imaging | • Quantum data representations retain the full quantum information from medical images, avoiding loss of detail from classical conversion – for example, quantum phase estimation can precisely characterize frequency spectra in MRI, enabling higher resolution and definition. • Quantum computing could assist in the analysis of medical images and improve image processing steps, such as edge detection, which may enable image-aided diagnosis by allowing quantum algorithms to identify certain features, such as subtle tissue abnormalities in early-stage cancer. | 1. Quantum phase estimation 2. Quantum annealing 3. Quantum machine learning (QML) | 1. Next-generation medical imaging platforms for refined disease visualization (for example, quantum MRD) 2. Improved image segmentation for radiologist-assisted diagnosis |
Quantum Sensors | • Quantum sensors have extreme sensitivity owing to quantum effects that enable unprecedented spatial and temporal resolution for biological imaging and tissue monitoring. • Direct interfacing of quantum sensors with quantum processors enables learning algorithms with provable quantum advantage. • Real-time analysis of quantum sensor data with quantum algorithms provides rapid insights into disease states. | 1. Nitrogen-vacancy diamond thermometry 2. Nitrogen-vacancy diamond nuclear magnetic resonance 3. Optically pumped magnetometry | 1. Real-time immunotherapy-response characterization through immunotargeted quantum sensors 2. Monitoring tumor metabolic activity in vivo using tumor-specific nanoscale quantum sensors |
The Quantum Sensors theme in Heath and Medicine has a role in medical diagnostics today. GQI’s Outlook Report on Quantum Sensors offers a chapter on Medical Diagnostics where you can read more details about their use. For those who are interested in a market timeline perspective, we see quantum sensors in Medical Diagnostics used today, via GQI’s Strategy Playbook. See the next Figure.
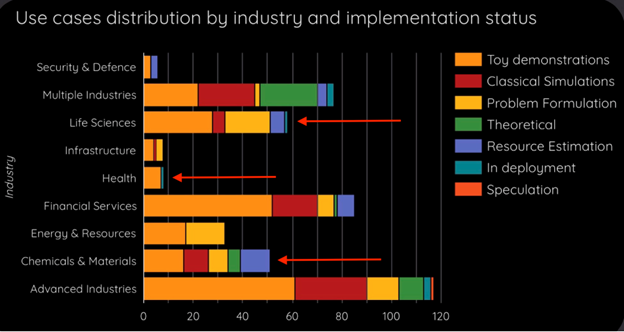
In Ramesh et al., 2024’s, Nature paper Table 1, the top three approaches: molecular modeling, genomics, and quantum imaging fit in today’s NISQ era, via hybrid classical-quantum computing. See QCR’s The Many Faces of Hybrid Classical-Quantum Computing: Part 1, Part 2, Part 3, where the quantum processor is a domain-specific accelerator. In Tomesh et al., 2024, they suggest that oncologists start working closely with domain experts to develop end-to-end hybrid applications, that apply quantum accelerators to certain processing bottlenecks and take overhead costs into consideration.
Cancer Applications: Specifically
More specifically, the Team’s “Quantum for Bio” work focusses on the discovery of biomarkers in multimodal cancer data. Biomarkers can be used to identify the onset or course of cancer. However, finding these biomarkers in such large, complex data is a challenge. Two computational issues arise:
- Accurate simulations for the exponential number of interactions within, or between, biologically linked, data modes, and
- the sparse characteristic dimension of numbers of patients.
About the sparse characteristic: the authors explain that, while each patient’s data spans gigapixel images and thousands of genomic features, the total number of patients is typically much smaller than the number of features, due to the time and expense involved in the data collection. This sparse characteristic makes it difficult for machine learning models to robustly analyze the data without overfitting. Cancer datasets are an interesting example where improved solution quality is more important than a quantum speed advantage.
The Team focuses on feature-selection, filter techniques to be involved in the downstream, learning task. These techniques employ a combinatorial optimization to choose a restricted subset of the data’s initial raw features, all the while maintaining interpretability. This step reduces the data’s dimensionality for data-scarce, machine learning techniques. Their blog article displays preliminary findings on how well hybrid quantum-classical feature selection algorithms perform in comparison to conventional baseline techniques. These are early research steps for quantum computing in cancer research, while displaying steady, incremental progress.
My own directories show similar incremental progress. If I ask SciSpace to provide answers to “What is the Unique Contribution to this Result?” for a dozen research papers which use quantum technology tools in cancer research, I see the following results. These “Unique Contribution” answers act as pseudo-Key Performance Indicators to show recent progress in this field.
(*) For those eager to explore the Strategy Playbook, GQI offers the ability to filter Use Cases, Value Chains, Geographies, and their Timelines. In the Figure, I selected those particular Health and Medicine Use Cases to see a Timeline for quantum sensors in Medical Diagnostics. GQI has other interactive Playbooks, as well. If you are interested, please don’t hesitate to contact info@global-qi.com.
September 4, 2024